JJQA: a Chinese QA dataset on the lyrics of JJ Lin's songs
Before the Beginning
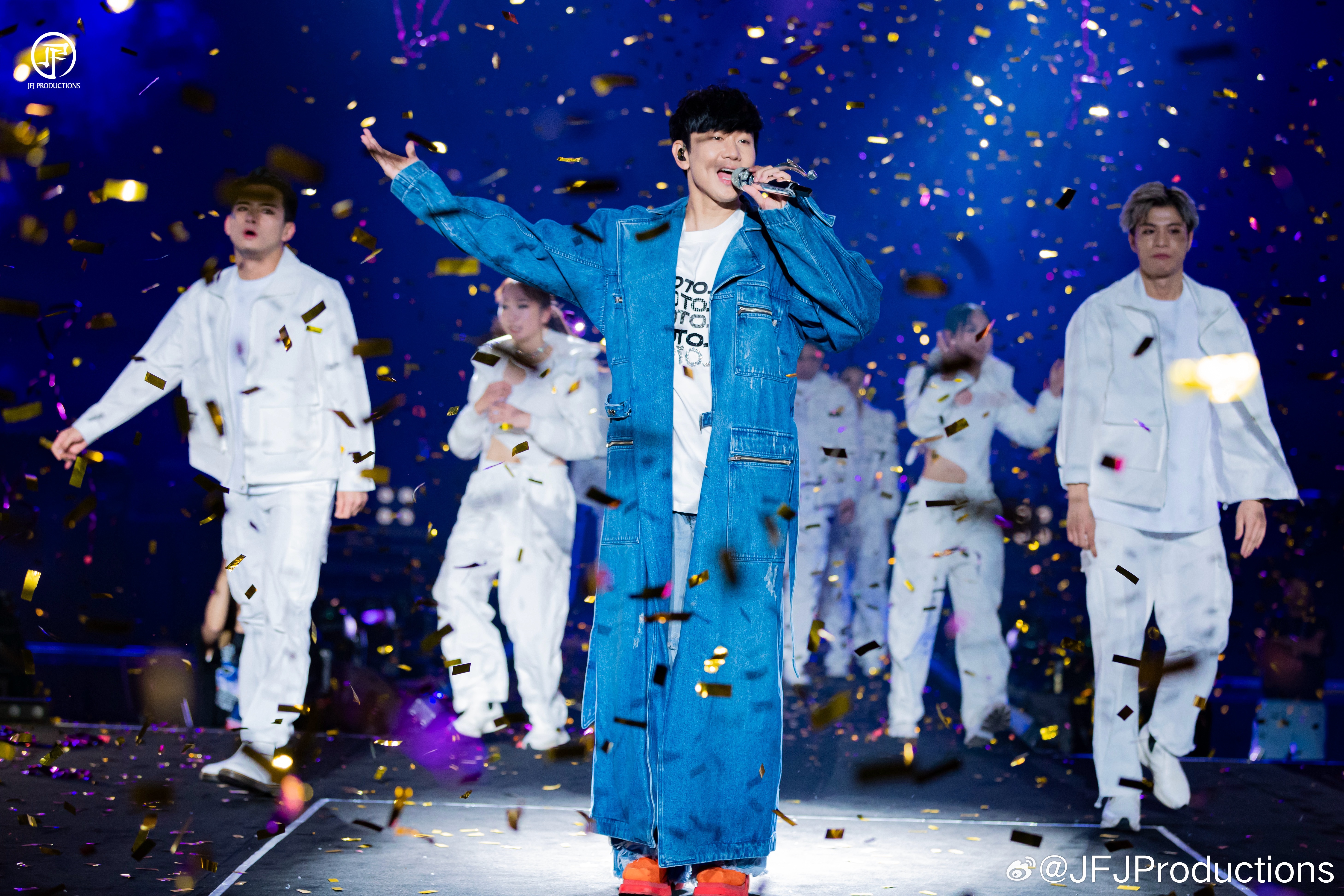
What? You really wonder where I was in the evening on 2023.10.29? I went to JJ Lin JJ20 World Tour in Xianyang😇. Though the position of my seat was not pretty good with the most expensive ticket😢, it was still a rather good experience. Hope that I would be at the first row in the next time🙃. Since JJ Lin is famous, it is not easy to get a ticket. With the "limited" help of my blank friends 🤣, we grabbed to buy my ticket in all of the three online platforms, but I would have failed without the only remaining luck🥳. Among the official platforms, I find that there is an interesting rule in the platform of JJ Lin, a APP called JJ20. It is that only if you are completely correct with 5 randomly selected one-choice questions about songs and experiences of JJ Lin, could you join the queue for tickets. In fact, instead of a senior fan, I just feel free to listen to some of his songs. Thus, the questions are terrible for me, let alone my pitiful friends😱. However, it is interesting that...... What about asking a large language model the questions on JJ Lin's songs?🧐
JJQA
here for the latest JJQA and better reading experience.
JJQA is now available in 🤗Huggingface. (click here)
NOTE: This is a sub-project of Open-Source-AI-Research, an experimental non-profit project focusing on AI research in an open source mode.
JJQA is under construction. Welcome to contribute.
Large Language Models (LLMs) have shown powerful capability of text understanding, analysis and generation. It seems a good tool for text-style knowledge based question answering (QA) where semantically retrieving related texts, understanding them and generating correct answers are required.
However, many feasible QA datasets are not challenging enough. First, given text-style knowledge might be easy to perceive and analyse. Second, the questions & answers follow commonsense. Thus, LLMs may benefit from training of language modeling and even take a shortcut. In this case, we want to build a new text-style knowledge based logical QA dataset where the text-style knowledge is tricky and LLMs are not likely to give correct answers without successfully retrieving and reasoning related texts.
Chinese is a language where each single character could contain abundant meanings while just a few words, especially pieces of lyrics, are able to express complex conceptions, feelings and impressions. Besides, Junjie Lin, known as JJ Lin, is a famous Singaporean Mandarin singer. The lyrics of his songs are always imaginative, poetic and romantic.
Hense, we propose JJQA, a Chinese text-style knowledge based question answering dataset on the lyrics of JJ Lin's songs, where related lyrics are provided as text-style knowledge for retrieval while the questions and answers are based on the lyrics. The Q&As are always abstract and follow anti-commonsense. For example, according to the related lyrics of a song called "爱情Yogurt", the question is "热量有什么作用?" ("What is the impact of heat?") and the answer is "降低爱情的过敏反应。" ("Ease the anaphylaxis of love"). It is indeed ridiculous and funny (you could find more in the dataset)🤪. Even human beings could not give the right answer without knowing the related lyrics. In addition, LLMs are not likely to naturally generate the right answer with the capability from training. Therefore, only if the related lyrics are retrieved and understood, are the right answers possibly generated By LLMs.
Dataset Details
JJQA is now available in 🤗Huggingface. (click here)
According to QQMusicSpider, we crawled lyrics of all songs of JJ Lin from QQMusic. After data cleaning and label annotation, 648 Q&As with 181 related song lyrics are included.
Three fields ("qa", "song", "song_index") are included in JJQA.
"qa" contains Q&As with 6 features. "q" and "a" are a question and the corresponding answer. "song_title" and "song_id" are the title and the corresponding id of the related song. "id" is the id for the Q&A. "rf" locates the lines of lyrics for reference, splited by a space " ".
"song" contains information of songs with 4 features. "title" and "name" are the title and the corresponding name of the song. "id" is the id of the song. "lyric" is the lyrics of the song, where each line is splited by "\n".
"song_index" contains one dictionary, whose keys are the ids of songs and values are indexes of the corresponding song in "song" field, to align QAs with the corresponding songs.
Baselines
We evaluate three baseline methods on JJQA. The first one (wo_info) is to "ask" the question directly without any additional lyric, which is to show the performance of uninformed LLMs; the second one (w_song) is to include whole lyrics of the related song as in-contexts; the third one (w_rf) is to just include related lyrics. w_song and w_rf are two reference lines for retrieval-based method.
Six feasible LLMs (ernie-turbo, chatglm2_6b_32k, qwen-turbo, baichuan2-7b-chat-v1, gpt-4, gpt-3.5-turbo) are included. We apply ernie-turbo and chatglm2_6b_32k in qianfan platform; qwen-turbo and baichuan2-7b-chat-v1 in dashscope platform; gpt-4 and gpt-3.5-turbo in openai platform.
We consider BERTScore with rescale_with_baseline=True as the metric.
The results are as follows.
LLM | Method | Precision | Recall | F1 | Date |
---|---|---|---|---|---|
ernie-turbo | wo_info | -0.0350 | 0.1568 | 0.0511 | 2023/11/06 |
ernie-turbo | w_song | 0.2472 | 0.5765 | 0.3895 | 2023/11/06 |
ernie-turbo | w_rf | 0.3600 | 0.6528 | 0.4864 | 2023/11/06 |
chatglm2_6b_32k | wo_info | 0.0466 | 0.1787 | 0.1066 | 2023/11/05 |
chatglm2_6b_32k | w_song | 0.2361 | 0.4606 | 0.3335 | 2023/11/05 |
chatglm2_6b_32k | w_rf | 0.4650 | 0.6477 | 0.5436 | 2023/11/05 |
qwen-turbo | wo_info | 0.2331 | 0.2150 | 0.2208 | 2023/11/05 |
qwen-turbo | w_song | 0.7673 | 0.8041 | 0.7804 | 2023/11/05 |
qwen-turbo | w_rf | 0.8600 | 0.8251 | 0.8386 | 2023/11/05 |
baichuan2-7b-chat-v1 | wo_info | 0.1755 | 0.2012 | 0.1857 | 2023/11/05 |
baichuan2-7b-chat-v1 | w_song | 0.4635 | 0.6324 | 0.5371 | 2023/11/05 |
baichuan2-7b-chat-v1 | w_rf | 0.6567 | 0.7272 | 0.6851 | 2023/11/05 |
gpt-3.5-turbo | wo_info | 0.2201 | 0.1983 | 0.2061 | 2023/11/06 |
gpt-3.5-turbo | w_song | 0.8031 | 0.7812 | 0.7884 | 2023/11/06 |
gpt-3.5-turbo | w_rf | 0.8110 | 0.7484 | 0.7758 | 2023/11/06 |
gpt-4 | wo_info | 0.2426 | 0.2377 | 0.2376 | 2023/11/06 |
gpt-4 | w_song | 0.8405 | 0.8587 | 0.8464 | 2023/11/06 |
gpt-4 | w_rf | 0.8865 | 0.8643 | 0.8732 | 2023/11/06 |
It is worth noting that Date stands for the time (UTC+8) for evaluation. In addition, a small number of samples are not feasible in the dashscope platform because of its safety system. We just skip these Q&As. (1 sample for qwen-turbo wo_info; 3 samples for qwen-turbo w_song; 3 samples for baichuan2-7b-chat-v1 w_song)
Discussion
We propose JJQA to evaluate the capability of a LLM on semantical retrieval and answer generation following contexts in Chinese. Further work could focus on how to implement effective semantical song/line-wise retrieval or enhanced answer generation. In addition, JJQA could be updated with Q&As of better quality or explanded to a larger dataset with Q&As on lyrics of different singers and even in different languages.
How to Contribute
First of all, please read the contribution terms carefully in Open-Source-AI-Research.
Second, fork this repository, make some improvements, add a record in Update Logs and just pull a request, which also means that you have already accepted the terms by default.
Thanks for your contribution!!!
Update Logs
2023_11_06 - bebetterest - bebetterest@outlook.com
DONE: initialized the first version of JJQA and baseline performance.
TODO: polish readme; add details on the annotation tool; open source related codes; rereview and update the dataset; explore some retrieval methods or generation enhancement methods...
Cite
Please cite this if your work is motivated from it.
@misc{JJQA,
title = {JJQA: a Chinese QA dataset on the lyrics of JJ Lin's songs},
author = {O.S.R.},
howpublished = {\url{https://github.com/bebetterest/JJQA}},
}